MIPL
Neuroimaging Applications

Neuroimaging analysis requires standardized data, thus the preprocessing of temporal and spatial varying MRI data is necessary. Our lab develops advanced methodologies regarding MRI data preprocessing including registration and segmentation
MRI preprocessing
Various kinds of medical imaging modalities exist and they provide distinct information. As the brain functions and structures are heterogeneous, using the information only from a single modality may not fully explain the characteristics of the brain. A combined model of multi-modality imaging might provide sufficient information for explaining complex brain function and structures. Additionally, the features from diverse imaging modalities could be used as good bio-markers of psychiatric disorders such as ADHD, autism, and depression as well as senile disorders such as Alzheimer’s disease and Parkinson’s disease.

Multi-modality data analysis
Radiomics Analysis
Radiomics analysis is the use of automated or semi-automated post-processing and analysis of large amounts of quantitative imaging features that can be derived from medical images. The rationale is that biomedical images contain information that reflects the various characteristic of tumor or regions of interest. Using medical imaging (i.e., computed tomography [CT], Magnetic Resonance imaging [MRI] and Positron emission tomography [PET]), the radiomics analysis may be useful for predicting prognosis and therapeutic response for various clinical scenarios.
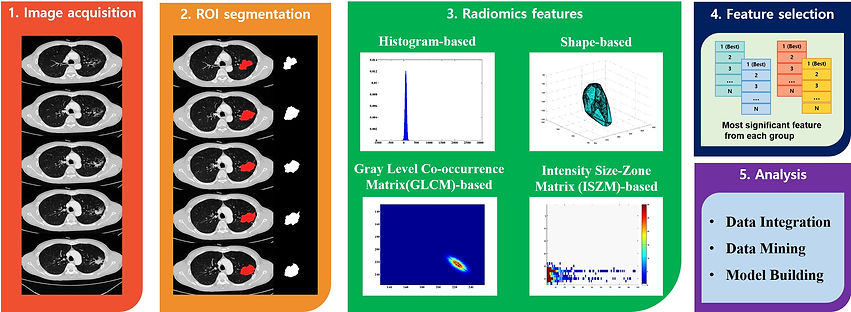
Imaging genetics Application
Combining information obtained from different modalities is becoming an important issue in modern biomedical signal processing. Imaging genetics is one of the novel fields of joint analysis, combining imaging and genetics information. Our laboratory develops advanced methodologies to identify brain networks or regions associated with genetic information specific for brain phenotype. For instance, Some multivariate methods, such as partial least squares regression (PLSR) and canonical correlation analysis (CCA) with L1-, or L2-regularization, have produced good results in extracting sparse features, ranging from gene expression level to brain networks.

Deep learning Applications
The deep learning, the dominant emerging technology of machine learning, has the potential to extract and learn features for various applications and has led to very high performances. The enhanced performance of deep learning might contribute to overcoming existing limitation of medical image analysis. We are researching advanced deep-learning methodologies for image processing, segmentation, classification, disease detection, and prediction of state in the medical imaging domain.
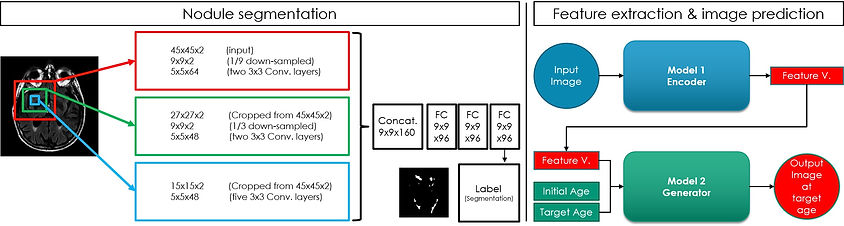